Why are there risks and setbacks in AI and ML algorithms for chronic disease and population health management?
1) Data is everywhere, yet not enough to pull off a quality algorithm.
a)Healthcare data is Messy
AI/ML algorithms require an enormous amount of data. However, the quality of data is equally important. An algorithm built on wacky data will produce a wacky output no matter the volume of data.
Based on this, healthcare data requires much “cleaning” to achieve any successful algorithm.
Presently, many of the developers of these algorithms are not willing to do the heavy lifting of “cleaning” the data well before using them to build algorithms.
b)Using Claims data for algorithms to predict clinical outcomes can be problematic
Insurance companies sit on troves of patients’ claims data. Using this data globally to predict patient outcomes like disease onset and the likelihood of future hospitalizations can be very tempting.
However, the problem with using such data is that it was collected ab initio for optimizing insurance processes like reimbursements and denials of service.
Their data collection was never meant to build algorithms to predict patient outcomes in the first place; as such, its output will be biased. The bias against minority patients happened in the Optum case-coordination algorithm because the algorithm uses health costs as a proxy for health needs.
Societally, we spend less money and resources on black and minority patients due to their less access to care because of systemic racism. Relying only on past spending [4] as a predictor of future expenditures and medical needs means skipping over sick patients who haven’t historically had access to health care.
c)Algorithms built on EHR data only are bound to fail
The electronic health records (EHR) adoption incentivized by the Affordable Care Act 2010 led to an explosion in electronic health data. Unfortunately, the data in our EHR is highly inaccurate[5]. An estimated 70% of the records in the EHR have the wrong information[6].
Our EHR is a giant billing machine whereby clinicians are hand-twisted to fill it with irrelevant information that doesn’t add value to direct patient care or the ability to use data for correct algorithmic prediction. Therefore, please exercise caution when using any algorithm built on EHR data.
d) Most data used to build algorithms are not representative
Due to the novelty of AI and the need for a lot of financial resources to run the data-heavy algorithms, most AI algorithms in the USA are built with data that are not nationally representative.
The geographical disparity of algorithm development is such that most data used to train health algorithms are from three states only: New York, California, and Massachusetts[7].
Considering the heterogeneous makeup of the US population, any of these algorithms will cause biased output and possible patient harm if implemented in other geographical locations where the algorithm data is not representative of the population.
Algorithmic performances change when deployed against different data sets. Healthcare algorithms are bound to be suboptimal because 70% of clinical algorithms are developed using data from only three states of NY, CA, and MA only.
Ahmed Digital -doc
Tweet
2)A lot of chaos from our Lack of consensus standards in health algorithm development and deployment
Due to the data explosion, many companies have now jumped into the race to gain market share in the offerings of social risk and stratification algorithms—these range from large insurance companies to small technology start-ups. Even data brokers now hawk their social risk models to anyone willing to buy[14].
These vendors have different sets of analytic tools, varying approaches, and levels of sophistication. The Lack of algorithmic standards becomes the bane of implementations. Add on the problem of Lack of explainability of the algorithm itself in addition to hiding the inner workings of their model based on intellectual property protection.
Many unanswered questions persist for auditing due to a lack of consensus best practice standards: which type of algorithm is best deployed for population health management and for which groups of patients?
3) The gatekeepers lack the strength and essential knowledge to keep the wacky algorithms out
There is an enormous knowledge gap in digital health among clinicians.
Many lack the fundamental technical insight to appraise an algorithm deployed for their patient care critically. Clinicians are supposed to ensure they are using the right tools for suitable patients and indications.
Add to this the problem of EHR-induced burnout[11] among clinicians, and you will see why this role of a trusted ally meant to critique every digital health tool implementation is far-fetched now.
4)Lack of adequate protective guardrails from Regulators
The most significant risk we face with AI/ML applications in healthcare is that vendors and AI developers are free to develop any algorithm without many regulations to protect patients from harm and bias. Unfortunately, our laws are lagging behind the speed of AI innovations, leaving us at the mercy of the industry to self-regulate.
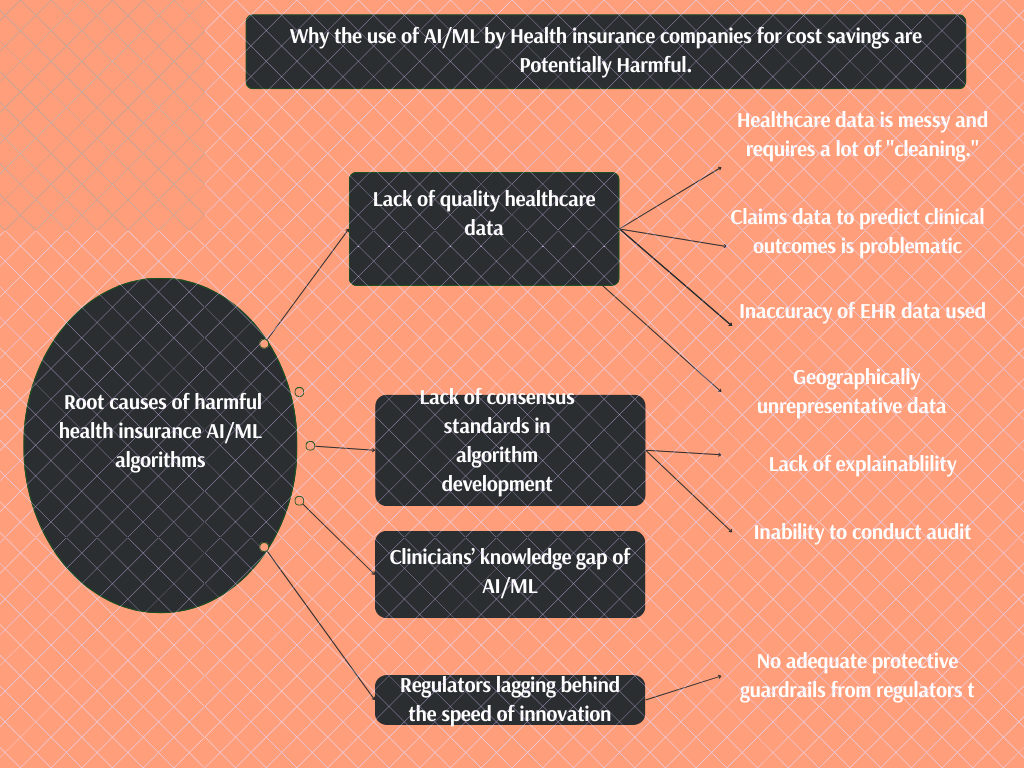
Digital health 360 degrees lens
Our electronic health record system is a giant “billing machine,” estimated to be 70% filled with wrong patient information. Yet, many algorithms are built on this data across the nation.
Ahmed digital-doc
Tweet
Despite AI/ML’s promising role in population health management and healthcare cost reduction, it is necessary to be wary of its unguarded application.
Our societal bias leading to health disparity finds its way into algorithmic outputs. The potential source of algorithmic harm spans the entire spectrum from problem selection and outcome definition to representative data availability and reliability.
The solutions to algorithmic bias are both systemic and technical. The technical solutions would not take us far, except if we uphold an ethically grounded system.
Our government and regulators must work towards reducing health disparity and systemic racism with the right policies. All stakeholders, including AI vendors and insurance companies, must take the concept of algorithmic vigilance seriously[8].
This process is similar to pharmacovigilance in the pharmaceutical industry and requires designing machine-learning models in ways that we can empirically examine after deployment[8].
It is well known that algorithmic performance changes when deploying it against different data sets, in various settings, and at other times. Consequently, the post-deployment audit should not be limited to the model output. Instead, it should examine whether impacts differ across relevant subgroups[9].
We should use relevant contextual information from the algorithmic surveillance activities to tweak the algorithm in an iterative feedback loop during the algorithm’s lifetime to prevent harm.
Adding SDOH to the data captured in the EHR can also reduce the bias from algorithms developed with EHR data[10]. However, it is equally vital that all clinicians learn to ask the right questions from algorithm developers trying to sell them a new tool.
Clinicians should ask at least two basic questions for their patients’ safety: How representative was the data used to develop the algorithm I am about to purchase? What is the plan for continuous algorithmic maintenance and model updating after deployment?