Exploring the nexus: the relationship between healthcare algorithmic bias and health Outcome
Healthcare algorithmic bias can result in heightened inequalities in health outcomes. However, this unfavorable state of health outputs may be unintentional, as the origins and sources of these biases may pre-date the conception and development of the algorithm[1].
Algorithmic bias in healthcare can be classified based on the algorithm’s complexity: simple algorithm bias vs. complex algorithm bias. The nature and impact of biased output from simple algorithms differ from that of complex models.
Algorithmic bias can also be classified based on the type of stakeholder implementing the algorithms. For example, the biased output can occur at the level of Payer-implemented algorithms or clinician algorithms at the level of direct patient care.
In addition, stakeholders affecting other social determinants of health can also apply biased algorithms.
In this write-up, we will analyze the various forms of algorithmic bias with contemporary instances where they occur.
Types/forms of Algorithmic bias
a) Algorithmic bias based on the level of Algorithmic Complexity;
-
Simple healthcare algorithmic bias
Simple healthcare algorithms existed long before the hype around big data-type algorithms of artificial intelligence and machine learning (AI and ML).
Clinicians use these simple algorithms, in the form of various scoring systems/calculators, to individualize treatments or clinical decisions at the level of direct patient care.
Most of these calculators involve “adjustment” for race or ethnicity in their output scores.
Unfortunately, these adjustments are based on outdated racial science or biased data showing a correlation and not causality between race and clinical outcomes[2]. In addition, recent advances in genomics have shown that race is not a reliable proxy for genetic differences.
Over the years, these racial adjustments in algorithms continue to erroneously prioritize race as the most critical factor in patient outcomes at the expense of the more important social determinants of health(SDoH).
“We continue to overrate the impact of genetics on disease outcomes at the expense of environmental factors and do not realize that an individual’s zip code is more critical than their gene code in health outcome.”
Simple algorithmic bias in clinical practice
- The eGFR calculator: The glomerular filtration rate (GFR) measures the kidney function’s performance. The higher the score, the better the kidney health.
Clinicians use it for determining the various interventions for patients with kidney problems: when to start dialysis or recommend a kidney transplant.
This scoring system adjusts for African-American patients, increasing the score by up to a factor of 1.2 higher than Caucasian patients with similar risk profiles.
This relatively higher value delays referral to specialist care and listing them for necessary kidney transplants[2].
Despite being four times more likely to have kidney failure, black people are placed at the bottom of the list for kidney transplants by algorithms[4]
- The VBAC calculator: The Vaginal Birth after Cesarean (VBAC) Risk Calculator estimates the probability of successful vaginal delivery after a prior cesarean section.
This calculator also adjusts for African Americans and Hispanics. This adjustment predicts a lower chance of success in people of color than in Caucasians of similar risk profiles.
This dissuades obstetricians from offering trials of labor to people of color, resulting in more unnecessary surgeries for people of color[2].
- The FRAX risk: This score calculates the 10-year risk of hip fracture in women with osteoporosis. The calculator also adjusts for minority patients, thereby producing a lower risk for people of color relative to a Caucasian woman of the same risk profile.
This relatively lower risk score in non-white patients may delay interventions in minority patients for osteoporosis therapy.
- The Emergency Severity Index (ESI): The ESI is the most commonly used emergency room(ER) triage calculator for determining the severity of patients presenting to the ER.
This algorithm assigns lower scores to black and Hispanic patients than white patients with the same illness severity [6,7].
This means blacks and Hispanics will receive less urgent treatment than white patients with the same severity of illness.
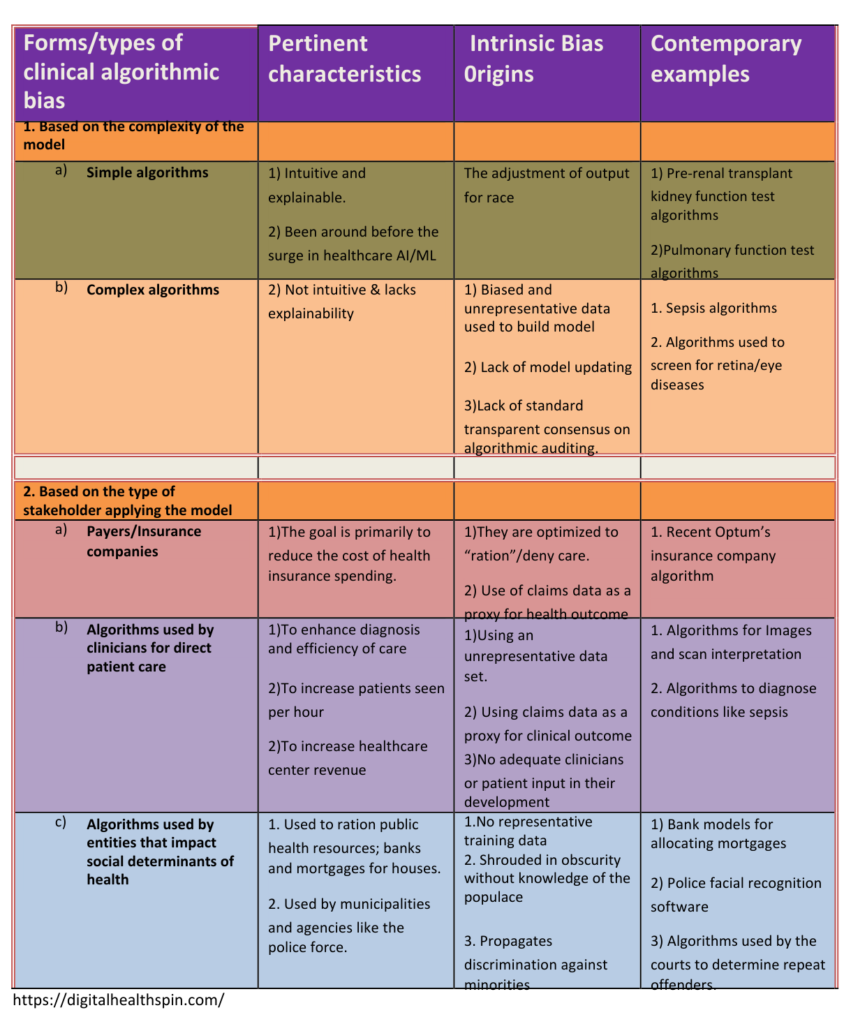
2. Complex Algorithmic Bias in Healthcare
Complex algorithms have many characteristics that make them prone to bias. An enormous dataset creates these giant models.
Additionally, some of them are complicated enough that the developers and clinicians may not have a logical explanation for how they arrive at their predictions(a black box phenomenon without explainability).
Sources of bias can originate anywhere along their development pipeline.
Although not as diffused as simple algorithms, these algorithms are gradually becoming ubiquitous in clinical practice. Insurance companies use them well for risk stratification and resource utilization.
Examples of complex algorithmic bias applications
- Dermatology A.I clinical decision support bias: Dermatological diseases appear amenable to AI image diagnosis of skin conditions like skin cancers. A recent thorough examination of some prominent AI tools to diagnose skin conditions showed they perform woefully in people of color compared to white skin[3].
The reason is primarily due to a lack of diversity in the training dataset used to develop the algorithm.
- Mechanical ventilation algorithms: A bias analysis of the algorithm used in deciding which patients will benefit from mechanical ventilation/critical care management in the hospital showed a significant variation in model suggestions across different ethnic groups [5].
Compared to Caucasian patients of the same severity of illness[5], Black and Hispanic patients were less likely to receive ventilation treatments.
- Pulse oximeter bias: Pulse oximeters are devices used to measure oxygen levels by placing them on the fingers. During the COVID-19 pandemic, these devices became more relevant as tools for self-screening and self-triage for folks with respiratory disease.
Unfortunately, they produce an overestimation of oxygen levels in people of color[8].
Essentially, it gives a false sense of higher oxygen levels in non-Caucasians with dire consequences of delaying lifesaving intervention as appropriate in people of color.
This bias stems from the fact that not enough people of color were included in building the pulse oximeter’s mechanism.
b) Algorithmic bias at the Stakeholder Level
-
Bias from payer algorithms;
Insurance companies’ harmful algorithms: Some insurance companies’ attempts to save costs with predictive models are rife with harmful predictions.
A recent example is Optum’s algorithm, which predicts patients with high future healthcare needs. The aim was to identify chronic disease patients/high-cost patients needing more support with transportation, home health aides, medication compliance assistance, etc.
The model turned out to discriminate against minority populations of the same disease severity as Caucasian patients[17]. Also, Cigna Health’s algorithm was indiscriminately denying care to older adults at an alarming proportion[9]
-
Bias from Clinician algorithm at the level of direct patient care
There are genuine concerns that breast cancer algorithms are worsening health disparity and reducing early detection in minority patients.
Our present reality is that the incidence of breast cancer and its mortality is higher amongst black women before the age of 45[10]. Add on the fact that the present screening age-based screening criteria for preventive mammography are also biased against minority populations[11].
These sinister realities get “backed” into breast cancer algorithms, resulting in a reduction in screening among minority women.
3. Biased algorithms from stakeholders who influence social determinants of health
Although these are not direct clinical care issues, they however have a direct multi-generational consequence on the health and well-being of minority populations in the long run.
Racial bias in housing: Historically, minority populations suffer from housing discrimination, from difficulty in obtaining mortgages to zoning laws. Present algorithm algorithms banks use to predict mortgage default discriminate against minority populations with the effect of unjust loan denials[12].
Justice system algorithmic discrimination: The use of predictive algorithms in the criminal justice system unfairly discriminates against minorities. One example is algorithmic prediction of persons to consider for bail, which usually discriminates against minorities[13].
Facial recognition software used in the criminal justice system falsely identifies minorities as criminals even when they are innocent[14].
The reason lies in the non-diverse data set, lacking adequate minority representation in training these algorithms.
Digital health 360 degrees lens
Simple and complex algorithms can exhibit dangerously biased output at different healthcare stakeholders, from payers to clinicians, at the level of direct patient care or public health situations like housing and incarceration.
These harmful outputs are mostly accidental, as most model developers I have encountered don’t wake up in the morning and decide what a bright day it is today to develop a biased, dangerous algorithm.
These outputs are a result of systemic racism and health inequity that sips into the models through biased data that lack representation.
This lack of representation is a consequence of lack of access to healthcare, amongst other socio-cultural reasons in minority populations.
Many of the present scoring systems used in clinical medicine to predict various outcomes are adjusted for race based on the wrong premise that race is the most critical variable that is responsible for our health outcomes.
Recent public health knowledge has debunked this assumption. These wrong genetic theories overamplify our human phenotypic differences at the expense of SDoH (like injustice, lack of access to healthcare, and the digital divide).
SdoH is more important than race in health outcomes[15]. Recent genomic studies show more variation within racial groups than between them[2].
Although this is an arduous issue, it is still solvable over time if we collectively acknowledge it and actively seek solutions to remedy algorithmic bias.